Statistician and data scientist continue to be ranked among the top jobs, most recently by U.S. News & World Report in 2017, which listed statistician as #1 on both its lists of Best Business Jobs and Best STEM Jobs, and Glassdoor in 2018, which had six analytics and data science jobs included in its 50 best jobs in America for 2018. We asked leaders in industry to answer the following set of questions to help students and statistics departments better prepare for jobs in the technology industry. We also hope the Q&A’s will help companies attract the statistical talent they desire.
Talkspace
Bonnie Ray currently leads data science efforts at Talkspace. Talkspace is a NYC-based startup that enables behavioral health care for all through providing a secure, affordable platform for messaging-based psychotherapy.
Number of employees: Talkspace currently has about 60 full-time employees, five of whom make up the data science team—part of the larger technology team that also includes software engineers and product managers.
Distribution of highest degrees: The backgrounds of the data science team members vary, with some transitioning into data science after having worked for several years in another technical discipline and others having just completed master’s or PhD degrees that included extensive data science training.
Growth (or lack thereof) in number of data scientists over last several years? The data science team was only created in 2017, but may grow by another one or two individuals over the course of 2018.
I completed a PhD in statistics from Columbia University and began my career as an academic, first as a postdoctoral fellow at the Naval Postgraduate School in Monterey, California, and then as an assistant/associate professor at the New Jersey Institute of Technology. During my time as a faculty member, I was consistently drawn to interdisciplinary work that had clear and tangible impact. I also came to realize standing in front of a class of undergraduate or master’s students to teach introductory statistics classes was somewhat nerve-racking for me, rather than fulfilling.
I decided to leave academia to take a position that allowed me to continue doing research while also providing opportunities for direct business impact. I took a position as a research staff member in the statistical forecasting group at IBM Research. As a research staff member, my responsibilities involved working directly with other IBM divisions to apply and/or develop new statistical methods to solve immediate business problems, while also filing patent disclosures and publishing applied research papers.
Over time, I took on responsibility for managing a small team of researchers and ultimately serving as director for an organization within research focused on development and application of AI algorithms to business challenges facing IBM and IBM’s clients. Moving into a management role required me to refine my business communication skills, as I was expected to present the work of my team to both internal and external clients on a regular basis, grow my strategic thinking skills to develop a solid research and funding roadmap for my team, and develop my people management skills to effectively motivate and provide feedback to my team. I also had to learn how to think more broadly, without necessarily understanding all the details of a research area.
More recently, as I’ve transitioned to the tech start-up world where I build and lead small teams of data scientists, I’ve needed to become comfortable with engineering concepts and agile development skills, while also continuing to hone my ability to translate business problems into defensible statistical approaches and skill at communicating statistical findings across different parts of the business.
What do you like about working in the start-up space? What are the challenges?
Working for an early-stage start-up allows me the opportunity to learn about all parts of the business, working directly with product managers to determine how data science can contribute to new product features, with the marketing team to understand the impact of advertising on customer acquisition and retention, with the clinical team to develop quantitative approaches to measure the quality of the service provided by therapists on the Talkspace platform, and with the commercial team to understand analysis and reporting needs of our commercial clients.
One challenge for me, personally, is the need to have a deeper understanding of the existing and potential computing infrastructure available to the data science team to ensure our data and computing needs are met. I’ve had to come up to speed on the various tool sets and platforms available for doing data science (e.g., Hadoop, Spark, Jupyter, GPUs, etc.) to successfully lobby for the needs of the team. Additionally, the team is sometimes asked to rapidly pivot from one priority to another, which can sometimes affect team morale—something I need to make sure I effectively motivate and manage.
How is the demand for statisticians in the technology industry? What are the main degrees you consider when looking for candidates with statistical expertise?
The demand for statisticians/data scientists in the start-up industry is currently huge, with newly minted graduates often starting at salaries 1.2x–1.5x that of new assistant professors.
While I do not look specifically for candidates with a degree in statistics when filling a data science role, I do look for evidence of basic statistical thinking and an understanding of fundamental statistical modeling approaches, such as linear modeling, survival analysis, and Bayesian techniques. Most of the candidates I look for at the bachelor’s and master’s levels have degrees in either statistics/data science or computer science (with a focus on machine learning and/or natural language processing). I also am open to candidates with degrees in electrical engineering, physics, computational biology, econometrics, and the quantitative social sciences—assuming they show a solid understanding of statistics fundamentals and appropriate coding skills.
What do you see as the most important statistical skills in the tech industry? What are the other important skills necessary for a successful career in this sector?
It is important that an individual have not only a firm grasp of core statistical modeling approaches such as covered in a strong master’s program, but also be competent enough to identify and understand appropriate statistical techniques s/he may not initially be familiar with to appropriately address the problem at hand. Solid programming skills are also necessary, with the specific language or tool set and the depth of technical knowledge dependent on the particular role. The most important skill needed for a successful career, however, is the ability to communicate effectively with colleagues across the business, both to understand the business needs for which data science expertise is needed and to communicate the results of analyses back to the business with minimal jargon.
What advice do you have for students interested in working in the technology industry? Any advice for students in general?
I would advise students to make sure their coding skills are more than competent, as working with any of the data sets typically collected will require effective and efficient data wrangling skills—and sometimes knowledge of multiprocessor, distributed, or GPU-based computing techniques.
I would also advise students to become comfortable with delivering the 80% solution (i.e., a solution for which an initial version can be developed quickly, but that may not address the complexity of the problem in its entirety).
Additionally, joining local data science–oriented meet-ups is really useful for establishing a network of colleagues from which one can learn and grow, as well as finding potential new career opportunities. Many individuals in the tech industry also maintain personal blogs or social media accounts focused on data science topics, which gives them exposure in the tech community.
What advice do you have for statistics and biostatistics departments (e.g., coursework, nontraditional training suggestions, research experience)?
I would encourage statistics and biostatistics departments to incorporate coursework on text analysis techniques, given the huge amount of information gathered in free text format today. I would also encourage exposure to additional computer science concepts, particularly those having to do with algorithmic complexity, as well as an overview of state-of-the art optimization approaches. Also, internships or work in the university statistical consulting center should be a required part of the curriculum to allow students to practice their problem definition, data wrangling, and communication skills.
What opportunities for advancement and professional growth exist for data scientists and statisticians in industry, and what advice for young professionals would you have to take advantage of those opportunities?
Advancement in the tech industry can be quite rapid, with individuals moving from individual contributors to team leads to chief data scientist roles in the course of only a few years, depending on the size of the company. I would suggest an individual think deeply about where he wants to take his career before taking on a management role, as often a move to management takes one away from further development of one’s core technical skills. However, working in the tech industry, particularly at a smaller company, often enables an individual to move into a role he may not have previously considered, such as a product management or business strategy role.
For career advancement in general, I would advise a young professional to be proactive in presenting his results to the business to gain exposure for his work, take advantage of opportunities to give talks at local data science community meet-ups, and/or participate in hackathons. These experiences provide broader exposure and networking opportunities that can lead to career advancement.
LinkedIn
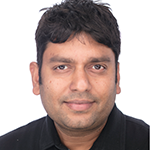
Number of employees: LinkedIn has more than 11,500 full-time employees, including more than 400 data scientists and more than 30 statisticians.
Distribution of highest degrees: ~350 PhDs, with many more employees who hold other graduate/postgraduate degrees
How many hires per year? More than 1,000 new hires per year
Growth (or lack thereof) in number of data scientists over last several years? 25% growth in data scientists last year
I earned a PhD in statistics from the University of Connecticut in 2001, with Alan Gelfand as my thesis adviser. My thesis involved fitting spatial models to large data obtained from disparate sources like satellite imagery, GIS, and census.
I got very interested in doing statistics for large data and joined the statistics department at AT&T Research Labs. After five fruitful years at AT&T, I decided to move to Yahoo! Research, where I became the chief statistician for the company. This was the best part of my technical career. I had the opportunity to create new statistical methodologies for large-scale problems that arise in consumer internet space. My work in this area had a significant impact on the business and resulted in several publications (including a book by Cambridge University Press).
After spending six years at Yahoo!, I decided to join LinkedIn in a management role. The last six years at LinkedIn have been the most fulfilling so far in terms of impact and job satisfaction. I lead a team of roughly 300 engineers and scientists who are responsible for all machine learning and statistical modeling at LinkedIn. The ability to use data and statistics to help connect talent with opportunity at scale is inspiring.
What do you like about working in the technology industry? What are the challenges?
I really like the broad impact one can have on the society and the agility with which one can accomplish things in the technology industry. The cycle from ideation to deployment is weeks, not months or years. Improving products through data-based algorithms is an integral part of the work. Statistical thinking and mindset is critical to success in this area. The volume and heterogeneity of data available to solve problems provide unparalleled opportunities to do novel methodological research. For instance, we changed the job recommendation algorithm last year to use random effects models. This improved the job application rates on the site by roughly 30%. The innovation was not so much the statistical model, but more scaling the estimation to billions of parameters and running such a large model to recommend jobs on the site. In addition to the technical innovation, that such work can create job opportunities for so many professionals on the planet is fulfilling.
While statisticians can have significant impact, the nature of the work is highly interdisciplinary. One has to collaborate closely with product management, engineering, security and privacy, legal, and others. Formulating the problem is often more of an issue and very challenging. Even when things get formulated, it can change quickly if there is a change in strategy or some new evidence emerges. Being adaptive in such a fast-paced environment can sometimes be challenging.
How is the demand for statisticians in the technology industry? What are the main degrees you consider when looking for candidates with statistical expertise?
Statisticians play a key role in many areas, and the demand for statistical expertise is growing at a rapid pace. Given the dearth of statisticians currently working in this industry, many in computer science and machine learning filled the gaps.
Success in areas like experimental design, causal analysis, and fraud prevention that is essential for almost every technology company today requires deep statistical expertise. In addition, large-scale statistical modeling is the core of all search and recommendation systems. We are interested in statisticians with both master’s and PhD degrees in statistics and a genuine interest in learning computational techniques that can help them apply statistics to large data.
What do you see as the most important statistical skills in the technology industry? What are the other important skills necessary for a successful career in this sector?
The most important statistical skill is, of course, a deep knowledge of statistical methods and the ability to solve practical problems. In addition, it is important to have a strong background in statistical computing and a genuine interest in learning new computational techniques such as distributed computing to apply statistical techniques to massive data.
It is also important to not just like, but enjoy, working in an interdisciplinary environment. Often, the most successful folks in the technology sector are those who gain a deep understanding of the entire end-to-end process over the years. Given how many opportunities exist to innovate using data-based algorithms, such individuals often end up becoming successful leaders and entrepreneurs.
The advent of cloud computing has made managing and computing with large data more of a commodity; the next big quest is to “commoditize” the extraction of intelligence (aka statistical inference) from data. There was never a better time for statisticians to join the technology sector than now.
What advice do you have for students interested in working in the technology industry? Any advice for students in general?
I have seen students with classical statistics training being a bit skeptical about joining the technology sector. They are not sure about the impact relative to others with background in computer science and machine learning. I would advise them to seriously consider a career in the technology sector. There is significant opportunity to make an impact and a high demand for their skills. Things that they may want consider are a) do they like technology in general; b) do they like doing applied work and enjoy working in an interdisciplinary environment; and c) do they enjoy learning new computational techniques to work with massive data. If the answer to all three is yes, they must consider a statistics career in the technology sector seriously. There is no better time to do so than now.
What advice do you have for statistics and biostatistics departments?
I would encourage statistics departments across the country to emphasize areas such as experimental design a lot more from both a theoretical and practical perspective. A bit more emphasis on modern computational paradigms like distributed computing with Hadoop/Spark would be useful. When teaching classical statistics, emphasizing what methodologies work well in different data scenarios (small data, large data) and a balanced portfolio of statistical inference techniques without over-emphasizing one particular area (e.g., only frequentist, only Bayesian) would be good. It is more important for students to develop strong statistical intuition and understand the pros and cons of different approaches. This would help them enormously in their day-to-day job when working in the technology sector.
Wellio
Sivan Aldor-Noiman works at Wellio. Wellio is a start-up of about a dozen people whose mission is to make it more convenient for people to eat healthier and better food, which they accomplish through the personalization of variety, healthiness, and cost, taking into account factors such as cooking ability, taste preference, health needs, and shopping preferences.
Emphasizing the importance of statistics and modeling, four members of the Wellio team are data scientists, with two having PhDs in statistics (including Aldor-Noiman), one a PhD in applied math, and another with a bachelor’s and master’s in computer science. There are also four software engineers.
Those interested in learning about Wellio’s internship opportunities should email Aldor-Noiman.
I was drawn to statistics as an undergrad at The Technion – Israel Institute of Technology, where statistics is housed in the industrial engineering department. After earning a master’s in statistics there, I did my PhD in statistics at the University of Pennsylvania Wharton School with Larry Brown and Bob Stine. I loved teaching, but knew I was not destined for academics and applied for jobs with technology companies.
I received several offers—including from well-known companies—but opted for the Climate Corporation, which was then a smaller, but growing, start-up working in the ag-tech sector. I chose them because of the wide-ranging statistical challenges I would have as one of their first statisticians doing modeling, including with spatial statistics and spatio-temporal dynamics. I also was really impressed with the impact and importance data science had in the product itself; it was clear this company wanted to use data science to its full extent. It was also quite clear to me that if I were to go to one of the more established companies with many other statisticians, the statistical challenges would have been narrower and professional advancement would have been much slower.
I spent five years with the Climate Corporation, an exciting period when it grew from 120 employees to more than 500 and was purchased by Monsanto. Early in my career there, I led a group doing weather modeling and risk insurance, which addressed many challenging and interesting problems statistically speaking (e.g., how weather impacts yields). I later started a remote sensing team (e.g., satellites, drones), which helped develop products that monitor crop development throughout the growing season. Both of these groups were extremely diverse and included statisticians, mathematicians, physicists, and remote sensing experts with mostly master’s and doctoral degrees.
I subsequently started two more teams. The first focused on fields experiments and observational studies, which unlike the small experiments Fisher conducted, were massive experiments across many soil and weather environments, agriculture practices, and crops. The second team focused on developing best practices for data science across the company. The challenges in this team quickly became more focused around culture development for helping data science grow.
Today, I lead the team of data scientists at Wellio. I really like both the mission we set for ourselves and the team. We are building food recommendation systems using advanced statistical and deep learning models to solve NLP and computer vision problems. This team pushes me to develop and improve my statistical, engineering, and leadership skills.
What do you like about working in the technology industry? What are the challenges?
I like the many hats I wear working in the technology industry, including statistician, data engineer, leader, and communicator of our findings. The latter presents a challenge in that one must also present the strengths, weaknesses, and limits of the results to clients and customers who want certainty. It’s hard for people to accept uncertainty; it’s a difficult concept. A data scientist must also understand what’s at stake for the customers. For example, when I was in the agriculture industry, a farmer’s livelihood was directly affected by our products.
The expectation of providing certainty has only increased with the recent emergence of artificial intelligence, which is perceived as almost having an air of magic. There is a great responsibility for data scientists to understand the limits of their models and yet produce and communicate something useful. One has to strike a balance between use and limitations, being careful that communication of the limits does not scare off the clients.
The other great satisfaction of working in industry is that I get to see the impact of my work with the consumer using the product. Getting feedback and improving the models and products brings endless technical challenges, which I really like.
How is the demand for people with statistics degrees in the technology industry? What are the main degrees you consider when looking for candidates with statistical expertise?
There is a big demand for statisticians across degree levels (bachelor’s, master’s, doctoral). The Bay Area in particular is a crazy bubble for statisticians. There is such huge demand here that statisticians are being pinged almost weekly by recruiters. Most of the data scientist positions are filled by people with degrees in statistics, applied math, computer science, economics, physics, or disciplines that use more applied statistics like remote sensing. Analyst positions are generally geared toward those with undergraduate degrees, though. As I discuss below, one has to be careful about job titles; they are often quite misleading.
What do you see as the most important statistical skills in the technology industry? What are the other important skills necessary for a successful career in this sector?
What differentiates those with statistics degrees from others is the ability to reason through an argument, the data, and the model and then communicate it. All these are fundamental skills of statisticians. We learn it in our first EDA class. You just can’t explain a model without these skills. When I haven’t hired someone, not having these skills is the main reason.
So many people don’t know where to start with a problem. When I am interviewing candidates, I ask them to look at the data and tell me what they see. I also assess their ability to build a model and assess its limitations, implicit in which are the questions: What is a model? What are the assumptions?
I also see an increased desire for candidates with causal inference expertise, with the technology industry starting to recognize anew that correlation is not causation. I know it sounds like old news for statisticians, but you would be surprised how people really look at results based on correlation and tell themselves the right story to fit their goals instead of developing the hypothesis in advance … like we are being taught in Stat101.
What advice do you have for students interested in working in the technology industry? Any advice for students in general?
I always provide the same advice: Go to a company looking for people to learn from, instead of a company where you would be the first data scientist and would have to teach statistics to everyone. In such a situation, you won’t learn as much about models and the many skills—technical and nontechnical—that will help advance your career over the long run.
It’s also important to ask what you are going to do and what the job looks like on a day-to-day basis. It might be they hire statisticians to produce dashboards and summary statistics, which may not be very challenging and therefore not helpful in the long term.
What advice do you have for statistics and biostatistics departments (e.g., coursework, nontraditional training suggestions, research experience)?
My beef is that some of them haven’t acknowledged that applied statistics is very important, just as is the need to understand theory. One must have balance between the two. Someone trained for industry needs to understand the theory and must be able to analyze data. Students must be exposed to real problems.
Taking classes in computer science and programming is also a very good thing. I had to learn programming on the side, and it’s harder for sure to do it this way. Furthermore, industry requirements for modeling and programming are very different than for statistical academia, which emphasizes statistical accuracy. Accuracy may not be sufficient in industry. Industry conditions can be much harsher. Sophisticated models may not be the first ones to try. A model that performs better from an engineering standpoint is likely the preferred one.
What opportunities for advancement and professional growth exist for data scientists and statisticians in industry, and what advice for young professionals would you have to take advantage of those opportunities?
Industry is still trying to figure out what data science is and what its career path is. Data analyst is often a junior position, but there is lots of variance. So, a senior analyst could be a PhD statistician. Don’t be tempted by titles, which can be almost meaningless. As I advised above, ask lots of questions about what the position will entail.
Those questions should also be about career path, but one shouldn’t be surprised if they struggle to answer the questions about career path. Two possible career paths are modeler-to-manager and statistician-to-principle data scientist. For the former, there is a big shortage generally of technical leaders who are good managers of people. More specifically for this audience, there is a big shortage of statisticians in the technology industry, but a much bigger shortage of statisticians who can manage.
Leave a Reply